A strategy for building successful experimentation programs
Leading digital experimentation tools such as Optimizely, Adobe Target, Google Optimize, Split IO, and VWO empower teams with limited statistical knowledge to run split tests and make informed decisions when designing new website experiences. This works great for organizations that are new to testing and can gain a lot from simple optimizations like updating call-to-action buttons or investing in more compelling images.
Unfortunately, organizations eventually run out of low-hanging fruit for experimentation. Easy incremental gains begin to shrink and so does return on investment from the testing program. Rather than just settling for smaller gains, we recommend you adopt our IDEA Methodology for A/B Testing.
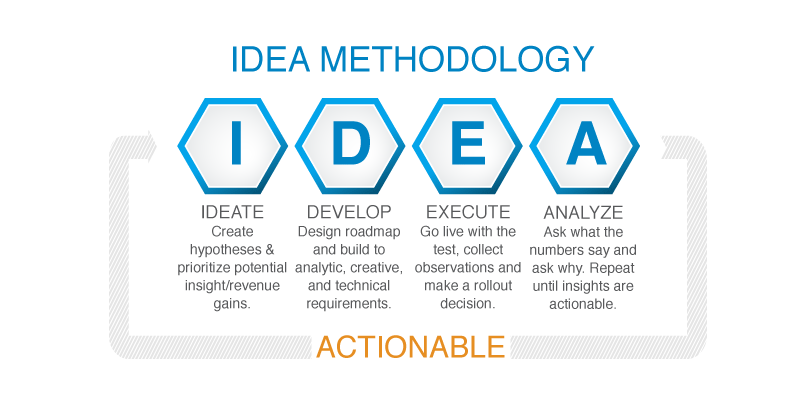
IDEATE: Creating hypotheses that answer business questions
The key to successful testing is good ideas, which lead to strong test hypotheses. There are three fundamental types of optimization experiments:
- Mitigate Risk: Implementing a best practice or backtesting a launched solution.
- Increase Revenue: Optimizing to increase purchases and profit.
- Reveal Insight: Experimenting to learn more about the consumer.
Most companies start testing with what we classify as risk mitigation. They test ideas already tried by other companies and accepted as industry best practice. There’s a good chance the variant recipe will win in these cases since the tactics have proven successful for others.
Typically, there’s no shortage of ideas to increase revenue. Marketers and analysts intuitively have projects and ideas to increase the performance of their products or services. Testing these ideas can be like gambling. If you test enough, it’s probable you’ll win sometimes, especially if you’re adept at understanding the odds and reading the situation.
In the last type of test, companies design experiments to reveal consumer insights. These insights can then be used to design additional tests, target marketing efforts, and optimize conversion flows.
DEVELOP: Ensuring great experiments
Once an organization has collected a repository of ideas, the next step is prioritizing experiments based on strategic objectives. A company must understand how each test will support strategic goals and what resources are available for testing. Once priorities are set, we’re ready to design the technical, analytical and creative requirements for each experiment.
By aligning early on a test’s key performance indicators, your marketing and analytics teams can ensure the analytics implementation is ready to track all the necessary data to judge test success. It’s best to invest this planning time in the beginning rather than realize you aren’t able to answer all of your pertinent business questions after a test is finished.
By forecasting the probable difference between the control and variant recipes, stakeholders can also align on the sample size necessary to judge an experiment’s success and the estimated test duration. One of the most common mistakes in A/B testing is allowing a test to run until it reaches significance, no matter the size of the lift.
When planning a test, it’s much more efficient to decide on the minimum statistical difference that can be considered a “win” and then run the test until you reach that sample size. When businesses fail to end tests sooner in order to reach significance on smaller gains, they fail to consider the opportunity cost of not running another experiment that could result in a larger win.
EXECUTE: Running successful A/B tests
Execution is the simplest phase of the A/B testing process, consisting of setting the test live and allowing it to collect observations.
The fundamental value of any testing tool is randomly splitting traffic once people trigger the rules that enter them into the test. Optimizely and Target are primarily frequentist. Optimize and VWO are primarily Bayesian. While some tools have advanced capabilities such as personalization, they all perform the same functions for basic testing, including splitting traffic, recording observations, and reporting results.
If the experiment was well developed, the stakeholders should have reasonable expectations on when they should see results, and there’s actually very little analysis required. Once sample size is reached, the test either wins or loses based on agreed upon KPIs and parameters.
ANALYZE: Dig into A/B Test data for deeper insight
You may ask yourself, if we already declared a test winner and made a rollout decision, what’s left to analyze?
Answer: Your consumers.
Now that we have a winning recipe, it’s time to ask what the numbers really tell us about our customers. Then we ask why. Wash, rinse, repeat.
If you ran an A/B.n or a multivariate test, now is the time to run ANOVA or regression to dissect the factors that seemingly perform best for various KPIs. You should also consider segmenting your consumers to see if specific audiences favored certain recipes or test factors. If this type of analysis is new to you, start by separating first-time visitors, frequent buyers, and everyone else. With such UX research methods, you may discover it’s beneficial to give first-time customers and loyal customers different experiences on your website.
If you’re testing marketing channels or a holistic customer journey, time series analysis could also prove beneficial. You can segment by test recipe or run a cluster analysis and create data-driven consumer segments.
The analysis possibilities during this stage are endless. In the same way that we prioritize experiments, it’s also important to prioritize the business questions we’re hoping to answer.
“People don’t buy visionary products, they buy solutions to their problems.” – Diana Kander, entrepreneur and bestselling author of “All in Startup”
Building on this idea, marketers and business executives don’t need analysis. They need answers to their business questions. Analysis is just a means to that end. When business stakeholders collaborate with analysts to successfully solve business problems, amazing things happen. The business gathers actionable consumer insights that can inform new test ideas and provide a competitive advantage.
IDEA: Building a better A/B testing process
The IDEA Methodology for A/B Testing and experimentation provides the structure and guidance necessary to improve an organization’s optimization practice. Companies that dig deeper to learn more about the actual human beings who use their products and services will continue to build competitive advantages over competitors that simply run experiments to optimize for broad audiences. If you’re ready to evolve your testing practice, let’s chat about it.