How testing pie recipes can teach your team about the human side of A/B testing
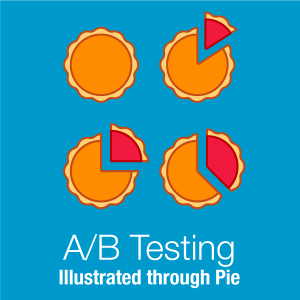
Non-mathematicians will be excited to find that A/B Testing requires a type of logic that goes beyond statistical calculations.
We’re testing people, and their behavior toward a recipe, not the recipe itself. It is tempting to take stock in the numbers alone or make behavioral insight leaps without accounting for alternative solutions. However, asking questions about what those numbers are really saying about your customers can save you from making costly mistakes and open doors to improved insights.
The Evolytics office recently illustrated some of the most common A/B testing critical thinking pitfalls while celebrating Pi Day.
Setting up an in-office A/B Test
To set up this test, we invited team members to bake (or buy) a pie for a taste test competition. We then created voting slips that aligned with our test brief measurement plan. The slips included our primary key performance indicator, willingness to purchase the pie, as well as secondary KPIs to monitor. We believed that our secondary KPIs would help us understand the motivations for pie purchase choice, and we could perhaps do a cluster analysis in order to create segments.
At noon, the office staff was invited to the kitchen to test our eight pie recipes. We allowed team members to stack the deck and vote multiple times if they wished and considered those votes as multiple devices, mirroring real-world visitor behavior.
We gave moderate instructions, but tried to answer questions somewhat vaguely. Remember, your customers can’t ask you questions when they get confused during an A/B test on your website!
What we learned about A/B Testing and humans
You don’t always know the motivations that drive your KPI.
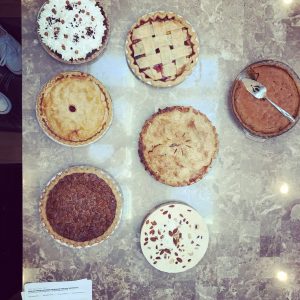
In our test, the winning recipe, defined as the recipe employees would be most willing to purchase, was a Pecan Pie. However, it did not win in either of our secondary metric categories that we believed would influence the decision. The takeaway for our A/B testing team is that we believe selling a Pecan Pie would be the most profitable, but we don’t know why. We do, however, know that deliciousness and health are not primary drivers of this behavior.
In future iterations, we could conduct a multivariate test to better understand the influence of various pie factors. We could also collect voice of the customer data to enhance the qualitative understanding of our consumers and inform a cluster analysis to find the best consumer segments to target.
Not all KPIs are created equally
In our test, we learned that there was little argument about which pie was the healthiest. Most employees voted for the Vegan/Paleo Snickers Pie with no added sugar. Since this pie was the most uncontested in a category, it would technically have received the highest score if we considered all KPIs equal. However, only 5% of tasters said they would purchase this pie.
This finding can impact both new and experienced A/B testing teams. As seasoned experimentation teams refine their A/B test reports to include secondary metrics, it’s important to give them appropriate weight, so factors with tiny influences are not considered equal to factors that truly drive decisions.
A lack of planning can lead to confounding variables
We allowed our bakers to really own the recipe they were testing. This meant they were allowed to market their pies and bring supplemental ingredients such as ice cream. The result? The bakers who brought homemade pies also brought notes about the ingredients. This may lead them onto getting the inspiration to buy themselves an Andrew James ice cream maker, so they can create these recipes and add-ons completely from scratch, adding to their appeal.
One of our analysts asked about the performance of homemade pies compared to store-bought pies because she said she couldn’t bring herself to vote for store-bought pies. Other analysts asked if it was fair to allow the product marketing from homemade pies because they felt those pies gained an unfair advantage.
Homemade pies performed better than store-bought pies, on average, getting more votes in at least one of the three KPI categories holistically. However, these were the same pies that put in extra effort with “advertising” through fun ingredient pie charts or healthy value propositions. Therefore, we have no idea which specific factors made these pies perform better.
In future iterations, we can use these confounding variables as factors in a multivariate test with multiple recipes that will clearly determine how much influence each factor has, or we could test each variable on its own in different A/B tests. For instance, testing two store-bought pies with and without a value proposition, then testing a homemade recipe against a store-bought recipe with marketing consistency.
Those tests who took advantage of our cross-device double voting rule did not stack the deck toward one pie
Not all observations are truly unique visitors, and humans aren’t particularly rational. They may have had perfectly valid reasons for voting differently each time. For instance, some voted for their favorite fruit-based pie and their favorite nut-based pie on each side of the card because “it really depends on my mood.”
Cross-device stitching can help us track “irrational” decisions such as this across users and devices, but the important insight is understanding that your test observations are humans with non-binary motivations and preferences.
Early test readouts aren’t reliable
With a quarter of the sample in, some stakeholders were curious how results were stacking up, so we did an early readout. With a quarter of the sample, there was a tie between two pies for our willingness to buy KPI, but the ultimate winner, the Pecan Pie, wasn’t even on our radar with a single observation choosing it.
While it’s tempting to read results early, it’s incredibly important not to put too much emphasis on early results. There are a lot of reasons that early results aren’t random or representative, but it’s easy for early results to get stuck in a stakeholder’s mind and create issues later when the data tells a different story. In future iterations, we may consider keeping the results hidden until pie recipes reach significance.
Your A/B test observations are humans, not just numbers
In summary, it’s important to remember that the observations in your test are humans. Their motivations change, and their behavior isn’t always as rational and predictable as we would like for it to be. However, with the right test setup and application of advanced statistical analysis, you can uncover real insights about your consumers that give you a strong competitive advantage.