A/B Testing is one of most impactful mechanisms for conversion rate optimization
In its simplest form A/B Testing is comparing one holistic website experience to another and measuring based on one key performance indicator (KPI). In academia, this is called hypothesis testing, and is commonly measured with a T-test. More advanced hypotheses may warrant multivariate testing. Ideally, an A/B Test does at least one of these three things for a business:
- Mitigate risk when introducing new features or redesigns
- Enhance understanding of consumer behavior
- Increase revenue with conversion rate optimization
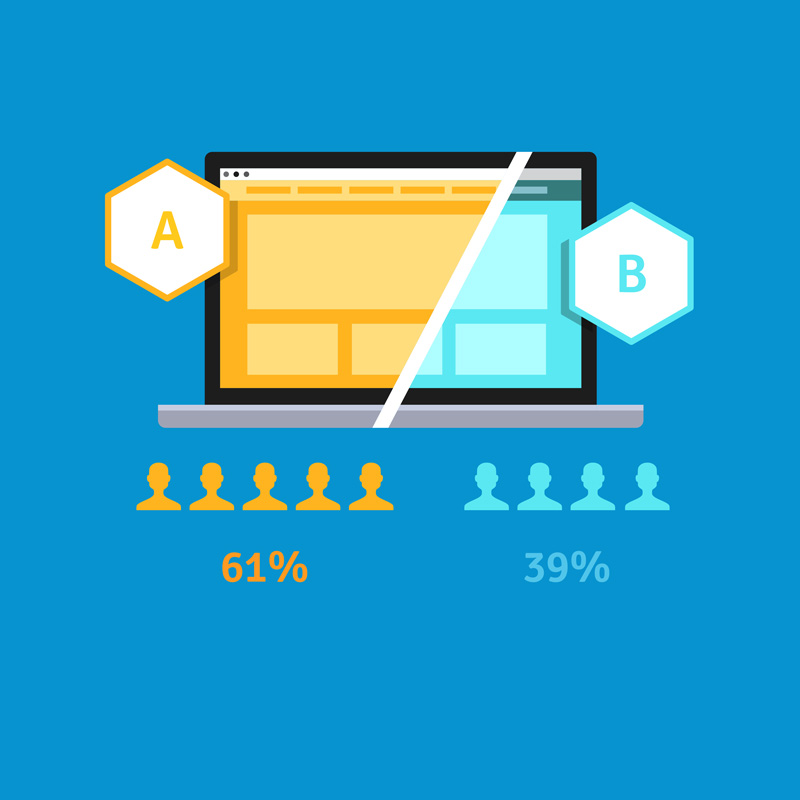
If, like me, you’re a fan of the book Everybody Lies by Seth Stephens-Davidowtiz or The Lean Startup by Eric Ries, you know that experiments serve as more reliable measures of customer satisfaction, opinion, and willingness to pay for a product or service than surveys alone.
Here are some basics to get you started.
A/B Testing begins with ideas
At Evolytics, we aim for data-driven ideation. If you review your customer journey, where are there issues in the buying funnel? Do you find that certain channels act far differently than others? What about devices or simple customer segments such as new and returning visitors? If you have voice-of-the-customer data, what is it telling you? As a user, what would you change about your website — can you find evidence in the data that you’re not alone? Do you have any upcoming big bets that need assumptions confirmed?
Once you have a list of potential ideas, begin prioritizing them. For the rest of this post, we’ll assume you’ve picked one.
Define your measure of success
The first step is deciding what you want to optimize. This may be time conversion, even revenue per transaction, product views, or bounce rate. You can essentially optimize anything that you can measure. We recommend choosing one true north KPI that will be the ultimate decision-making variable.
You can add a variety of secondary KPI’s, those that you want to ensure you do no harm to. For instance, if your primary KPI is product upgrades, you may want to monitor conversion rate and revenue per visitor as secondary KPI’s to ensure you don’t harm overall revenue by increasing revenue per order.
Finally, you should also monitor behavioral KPI’s. These are directional indicators that help us understand why an experiment won. Behavioral KPI’s also help us develop personalization tactics and continuous iterations.
Once you identify your KPI’s, determine the minimal detectable lift (MDL). This is usually driven by statistical and business needs. For example, if you want to test a more expensive new login widget, you may need to see a sign-in lift of at least 3% to break even on the technology investment. Additionally, you may find that if you want to have statistically significant results prior to your next quarterly share out, you’ll need a 6% lift based on the available traffic to build your sample size. Of course, knowing your MDL doesn’t make it realistic, understanding typical lifts and variation levels will help you determine what’s realistic.
Turn your ideas into hypotheses
While writing out your hypothesis may not seem that important, it will help you interpret your results later.
A hypothesis statement is a combination of your idea and your MDL. You can always answer it with a yes or no, and it’s easiest to make it an if-then statement.
- If we leverage the premium authentication widget, then we will increase logins by 6%
- Variant – Control > 6%
Hypothesis tests in statistics are built around trying to disprove the null hypothesis. This makes the hypothesis above (the one you want to be true) the alternative hypothesis. The null hypothesis includes an equality. In this case, the null hypothesis is that the difference between Test and Control is equal, or at the very least, not 6%. The final hypothesis looks like this:
- H0: B – A > 6%
- H1: B – A > 6%
Since A/B testing relies on samples, you will want to verify your results are statistically significant.
It is always better to test two experiences at once with randomly assigned visitors rather than simply making changes and testing to see if the measurement improves. Why? Concurrent testing with random assignments allows for added validity – when interpreting the results, you can have peace of mind that nothing else except the website changes are affecting the results. Pre-Post tests, are sometimes a reality, but you can never quite ensure correlation is causation with a pre-post when you can’t control for outside market factors.
What steps have we covered so far?
- Identifying a measurement to optimize
- Deciding upon a success metric
- Developing a hypothesis test
Read A/B Testing Basics for Website Optimization: Part 2 for how to interpret the results of a T-Test in Excel, but breathe easy, many digital testing tools do this sort of heavy lifting for you.