Changing the testing recommendation for asymptomatic people impacts Covid-19 KPIs and response measurement
C.D.C. Now Says People Without Covid-19 Symptoms Do Not Need Testing
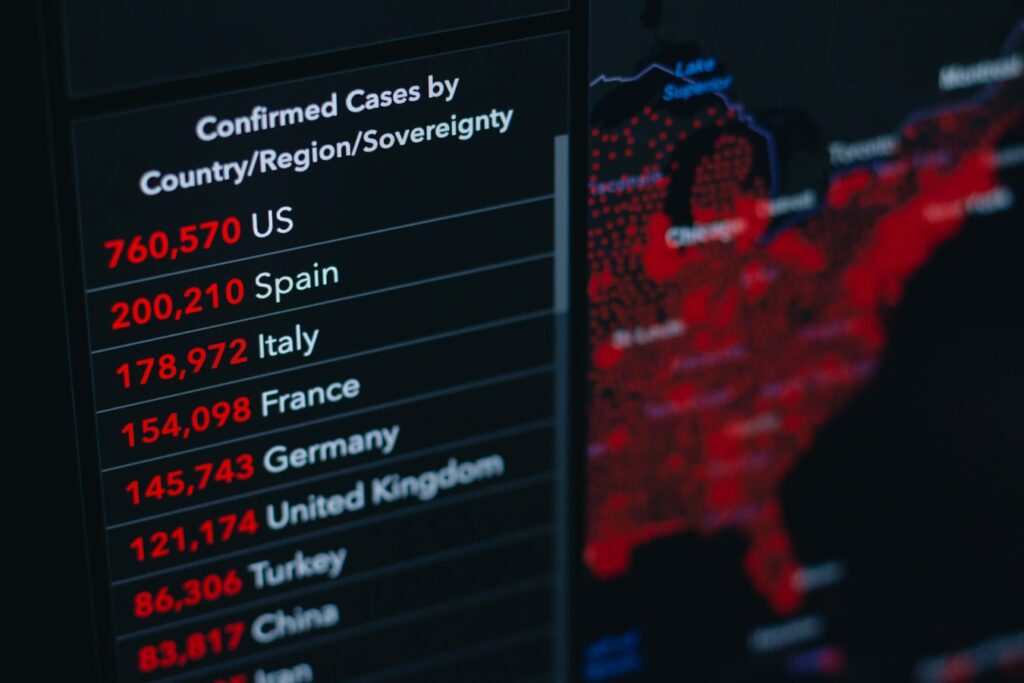
Katherine J. Wu‘s recent article explaining how the US government wants to decrease the amount of tests conducted amid a global pandemic raised questions. As an analyst and US citizen, I’m concerned about these new guidelines from the Centers for Disease Control. By allowing asymptomatic people to go untested, we are changing the underlying logic we use to calculate the infection rate and mortality rate of Covid-19. Will this change spread more misinformation and confusion about the coronavirus response? Now might be a good idea to be looking at insurify reviews to find the best health insurance possible as it doesn’t seem like the government has a handle on the situation. It might be better to take your health into your own hands.
Don’t Make Assumptions with KPI Logic
I would argue that if you asked a room full of analysts for the infection rate or mortality rate of a given city and gave them the same raw data, you risk a roomful of different answers.
While arithmetic is not subjective, the logic behind it can be.
Calculating the Covid-19 Infection Rate and Mortality Rate
If we’re defining rates, we must answer a number of logic questions before we decide the proper numerators and denominators.
Is it the number of positive cases divided by the number of tests given? Is it the number of positive cases divided by the city’s population? How do we find the nearest testing centre? Do we simply search using keywords like “antibody testing boulder” “covid testing centre Denver”, and so on? How do we solve for asymptomatic carriers who are not getting tested, selection bias associated with voluntary testing, re-testing, or test accuracy rates? When and how do we consider a case resolved and move a positive case to the “healthy” column? If I live in Missouri, but get a positive test in a Kansas hospital, which state(s) should claim the positive test? If I get tested three times, do I count as one test or three? Should those who are asymptomatic count as infected?
As you can see, there are several possible combinations of numerators and denominators, and while there are arguably many “right” answers, consistency is key.
Looking at an In-Depth Example
Calculating the Covid-19 Mortality Rate
Now, let’s hone in on specific ways we could calculate a mortality rate. Even when all intentions are good, misunderstandings can have the same dire consequences as misinformation.
Our Evolytics team came up with four ways we would potentially calculate a Covid-19 mortality rate.
Covid-19 Deaths / Total Population
The benefit of this method is comparatively trustworthy, reliable numbers. Few deaths fly under the radar, and populations are also fairly well-known. It’s also a solid bottom-line metric to measure the joint impact of social distancing policy and medical treatment, since it measures the entirety of the Covid-19 journey – from all citizens regardless of infection to the final, undesired outcome we are trying to reduce.
However, this may not fully answer the fears of healthcare workers and at-risk citizens, who might ask: what is my chance of death if I contract Covid-19? Exactly what gamble am I taking when I leave my house? Any helpful hints that they can gather at this point, either through dedicated medical social network platforms (like Sermo), or other trusted medical sources, could be of great value to them.
There are certainly other factors to consider from a mathematical standpoint. How do we handle migration? For example, approximately 7,000 Stanford University students are returning this fall to Stanford, CA, which according to the 2010 census, has a population of just under 14,000. If we add those 7,000 students to the Stanford, CA population, the mortality rate will decline in the immediate time frame, but it’s certainly not because of improved medical treatment or prevention. The real reason is the influx of a large, low-risk population.
Covid-19 Deaths / Tests Performed
In a perfect world, this would show us the mortality rate for those exposed to the virus, giving citizens and doctors a clear risk indicator early in the process of treatment. Unfortunately, we do not live in a perfect world where everyone is tested equally upon exposure. Some individuals are tested at frequent intervals, others must jump through hoops to be tested, and some don’t see it as important or necessary.
Furthermore, testing protocols have changed with time as tests have become more widely available and more is known about coronavirus. This makes a time series analysis, or even a basic trendline, using this metric impossible because the denominator (the number of people getting tested) is inconsistent and will show false swings in the data, giving the impression that things may be getting better or worse when, in fact, nothing may have changed. Based on this metric, the mortality rate may jump substantially if the recommendation to stop asymptomatic testing is adopted, since the denominator would shrink without corresponding changes to the numerator.
From a logical perspective, if a person can only meaningfully die once, should we also deduplicate their number of tests? Meaning, if someone was tested five times, should they count as five tests performed or one? If we say five tests = five tests, then we must know that the mortality rate could never be 100% even if, theoretically, the death per contraction rate were 100%. Additionally, it might give a false sense of security, since it would deflate the percentage by increasing the denominator – which brings us to our next potential calculation.
Covid-19 Deaths / Positive Cases
The benefit of this calculation for mortality rate is that it shows the fatality rate of contracting Covid-19, and more accurately measures enhancements in medical treatment, and corresponding danger to patients.
The major drawback, of course, would be that the number of positive cases is not entirely reliable, especially if we stop testing asymptomatic people. Like the Covid-19 Deaths / Tests Performed metric, Deaths / Positive Cases might falsely indicate a spike under the new reduced testing guidelines. If we were to expand testing and say, everyone returning to work or school must be tested weekly, then we would likely see a large reduction in the mortality rate, but a corresponding increase in the infection rate. Since the asymptomatic infection rate is unknown, we really cannot estimate the potential impact of such a move.
If we use positive cases as the denominator, how do we account for the potentially (depending on the specific test performed) high false positive and false negative rates?
Covid-19 Deaths / Resolved Cases
An evolution of Deaths / Positive Cases, this measure more accurately represents the fatality rate of contracting Covid-19 because it doesn’t allow new cases to inflate the denominator. It keeps new, unresolved cases in limbo until someone has either recovered or passed away.
This metric may have a long delay as patients recover, meaning it is a lagging indicator, a potential problem for something that has thus far warranted frequent monitoring. Like the other metrics that rely on testing for the denominator, the number is also prone to inconsistency as it depends on changing testing guidelines and protocols.
Why Covid-19 Metrics Should Matter to Voters
Covid-19 should matter specifically to voters because it’s so easy for politicians, scientists, healthcare workers — and even relatives you wish you were more socially distant from — to misinform with numbers. Is every news network and municipality using the same logic when reporting on mortality rates? If you see mortality rates changing over time, could it be due to underlying logic changes? Are there so many gaps in data that a benchmark of a “good” response is even possible to find?
How can we hold our elected officials accountable for a response we cannot properly measure?
Data-Informed Voter Series
The Data-Informed Voter Series is a 2020 passion project for a team of Evolytics analysts. We aim to be as politically neutral as possible while discussing the data, implications, and interpretations we see in the news. We discuss topics as a pseudo-editorial board with the aim of informing voters on how a professional analyst would interpret data during an election cycle. This project team consists of John Carney, Jay Farias, Liam Huffman, Brian Johnson, Anoush Kabalyan, Laura Sutter, and Krissy Tripp.
Related Data Stories Blog Posts
Data stories from the experts.
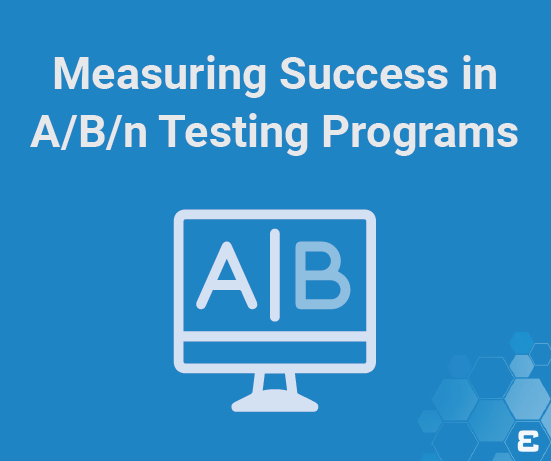
Measuring Success in A/B/n Testing Programs
The Whole is Greater than the Sum of its Parts The results of your latest A/B/n test are in-hand, they’re…
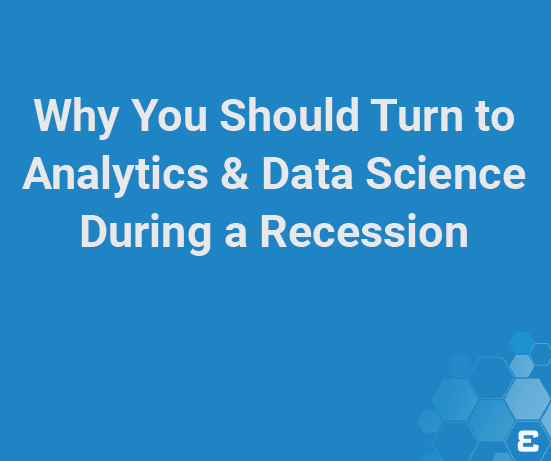